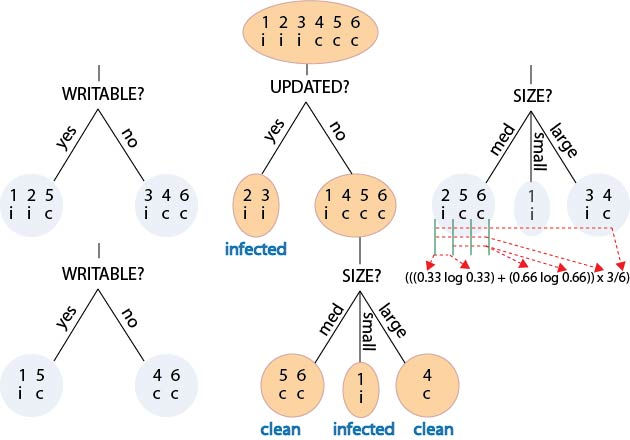
Decision Trees Crack Download This learning application features a training set, test set and a tree builder. Each example in the datasets can be viewed, moved to or out of the training and test sets. The tree builder and the tree itself are also easily manipulated. After viewing the tree, the testing can begin, with the results being displayed in the test set. For more information about this applet please refer to the main page for more information: Learn! Decision Trees Torrent Download with Apriori is a tutorial applet demonstrating how to use a decision tree for real-world applications. The applet features: (1) A tutorial - which takes the student through the fundamental concepts of Decision Trees Serial Key. (2) Example datasets and a complete implementation for teaching and illustrating Decision Trees Crack Mac. (3) A gallery of 12 advanced tutorials with implementation details and source code. (4) A Problem Solver, which can be used to identify the appropriate number of decision tree leaves, and the appropriate classification criteria for training examples, for a given problem. (5) Support for sorting and searching datasets on the basis of their column or feature values. (6) Test data sets, which can be used to evaluate the performance of a trained decision tree. (7) Algorithms and heuristics for building decision trees. (8) An example implementation of the C4.5 algorithm for building decision trees. (9) A discussion forum, where problems and questions can be posted and answered. (10) A command line interface for the applet which can be used to create test data sets for a given problem. This is the sequel to the Learn! Decision Trees tutorial applet. In it, the student learns how to implement a bootstrap aggregation decision tree. The applet features: (1) A tutorial - which takes the student through the fundamental concepts of bootstrap aggregation decision trees. (2) Example datasets and a complete implementation for teaching and illustrating decision trees. (3) A gallery of 12 advanced tutorials with implementation details and source code. (4) A Problem Solver, which can be used to identify the appropriate number of decision tree leaves, and the appropriate classification criteria for training examples, for a given problem. (5) Support for sorting and searching datasets on the basis of their column or feature values. (6) Algorithms and heuristics Decision Trees Crack + Full Product Key Free [Updated-2022] The KEYMACRO applet allows you to create, save and view your own datasets. The applet provides two modes: the training set mode and the test set mode. Once a dataset is created in the training set mode, it can be copied to the test set and vice versa. The training set can be used to train a decision tree to classify the test examples. The applet shows a simple one-layer decision tree to learn and classify your dataset using the decision tree. The applet's Create Mode allows you to view and manipulate the dataset, and the Training Set mode provides tools to build the decision tree. When building a decision tree manually, you can view all the examples in your dataset to gain more information that can guide your decisions. When the tree is built, you can test the tree against the test examples to learn the classification performance. The KEYMACRO applet allows you to create, save and view your own datasets. The applet provides two modes: the training set mode and the test set mode. Once a dataset is created in the training set mode, it can be copied to the test set and vice versa. The training set can be used to train a decision tree to classify the test examples. The applet shows a simple one-layer decision tree to learn and classify your dataset using the decision tree. The applet's Create Mode allows you to view and manipulate the dataset, and the Training Set mode provides tools to build the decision tree. When building a decision tree manually, you can view all the examples in your dataset to gain more information that can guide your decisions. When the tree is built, you can test the tree against the test examples to learn the classification performance. The KEYMACRO applet allows you to create, save and view your own datasets. The applet provides two modes: the training set mode and the test set mode. Once a dataset is created in the training set mode, it can be copied to the test set and vice versa. The training set can be used to train a decision tree to classify the test examples. The applet shows a simple one-layer decision tree to learn and classify your dataset using the decision tree. The applet's Create Mode allows you to view and manipulate the dataset, and the Training Set mode provides tools to build the decision tree. When building a decision tree manually, you can view all the examples in your dataset to gain more information that can guide your decisions. When the tree is built, you can test the tree against 77a5ca646e Decision Trees Crack+ [Win/Mac] Decision Trees applet is a feature of the Physics of Events applet and the Buckyballs applet. Legacy Games Category:Business simulation games Category:Windows games Category:Amusement park simulation games Category:Software that uses Scintilla Category:Puzzle video games[The role of B-vitamins in the management of a young diabetic patient]. The etiology and pathogenesis of diabetes mellitus (DM) are multifactorial. Despite this, there is a very important unmet medical need in regard to DM. The search for new therapeutic approaches for DM is a complex task due to the diversity of pathogenic mechanisms involved. The management of DM has also become more complex because of the increasing number of drugs being used in treatment. As a result of these factors, the management of DM is often frustrating for patients, who are often faced with a diverse number of medications and often need several medical consultations to achieve optimal glycemic control. In this context, nutritional intervention is an important therapeutic tool to achieve the best clinical outcomes. The present paper gives an account of the role of B-vitamins in the management of DM.Q: UIImage imageNamed not working with base64-encoded UIImage I'm creating UIImages for a project of mine. The problem is, I get a crash the first time I try to load an image, like so: +(UIImage *)imageFromBase64String:(NSString *)base64String { NSData *imageData = [base64String dataUsingEncoding:NSASCIIStringEncoding]; UIImage *image = [UIImage imageWithData:imageData]; return image; } If I step through this code, I get to the return statement, and I can inspect the base64String variable as expected. The crash happens when I try to get the image from the UIImage. I'm not sure what's going on. I also tried putting the following code in an additional method and having it call another method to create a base64 string: -(NSString *)imageToBase64String { NSData *imageData = UIImageJPEGRepresentation(self, 0.7); return [[[NSString alloc] initWithData:imageData encoding:NSASCIIStringEncoding] autorelease]; What's New in the Decision Trees? This application demonstrates the use of decision trees. You can learn how to build a decision tree using several sample datasets. You can also view and manipulate the dataset before building a tree. After building the tree, you can test the tree against unseen examples in a test dataset. Note: The applet is restricted to the number of records in a dataset. To build a decision tree with more records than the available dataset, you can view the dataset in several datasets mode, delete the dataset, and then build the tree with the remaining dataset. Credits: This applet is a Copyrighted material and is distributed under the GNU GENERAL PUBLIC LICENSE version 2.0. Use it at your own risk. Please give proper credits to its author. 0 . C a l c u l a t e t h e h i g h e s t c o m m o n d i v i s o r o f h a n d q . 4 S u p p o s e - 5 * x + 3 2 8 = - 3 * b , 5 * x = - 4 * b - 0 * b + 3 3 6 . L e t f b e x / ( - 6 ) * ( - 4 - 2 ) . W h a t i s t h e g r e a t e s t c o m m o n d i v i s o r o f f a n d 2 4 ? 1 2 L e t l b e ( 4 2 4 / ( - 6 ) ) / ( ( - 4 ) / 6 ) . S System Requirements For Decision Trees: Windows XP/Vista/7/8 (32-bit or 64-bit) and Mac OS X 10.6 Snow Leopard. Nvidia GeForce 6xx/7xx/8xx, ATI Radeon HD 38xx or Intel HD Integrated Graphics GPU. 2GB RAM and at least 300 MB of RAM. 1.8 GHz Processor 512 MB RAM 300 MB free hard disk space Screen resolution at least 800 x 600 and 1024 x 768 pixels. OpenGL 2.0
Related links:
https://lulugipp2.wixsite.com/thioutranlerbco/post/fluxtime-studio-crack-for-pc
https://housedisk.com/wp-content/uploads/2022/06/MusicLab_RealLPC.pdf
https://www.raven-guard.info/flashcardinator-crack-with-license-key/
https://innovia-lab.it/wp-content/uploads/2022/06/karlat.pdf
https://gabonbiota.org/portal/checklists/checklist.php?clid=3830
Comments